Historical Trends in Agricultural Commodity Prices
Understanding historical trends in agricultural commodity prices offers valuable insights for predicting future movements. Here’s an exploration of key drivers and notable patterns over decades.
Key Drivers of Price Changes
Several factors drive agricultural commodity prices. Climate conditions, such as droughts and floods, disrupt production levels and create supply shortages, causing prices to spike.
Technological advancements lead to higher yields and efficiency, impacting supply and putting downward pressure on prices. Geopolitical tensions introduce volatility by affecting trade policies and tariffs, influencing global supply chains. Policy changes, including subsidies and trade agreements, can also alter market dynamics, affecting pricing.
Notable Patterns Over Decades
Examining price patterns over decades reveals some consistent trends. The 1970s experienced sharp price increases due to geopolitical events like the oil crisis, which raised production costs.
The 1980s saw stabilization as technological advancements in agriculture began to bear fruit. The 2000s witnessed price volatility with the advent of biofuels, which increased demand for crops like corn.
More recently, climate change has led to more unpredictable patterns, with extreme weather events causing significant fluctuations. This historical context helps in understanding and anticipating future trends in agricultural commodity prices.
Current Market Analysis
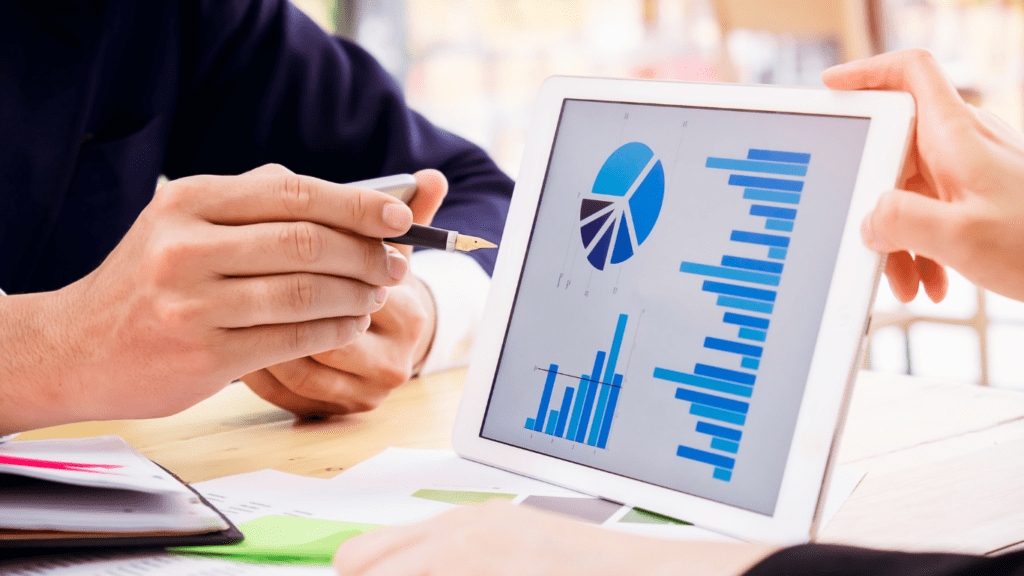
Observing current market trends reveals significant shifts in agricultural commodity prices. Key factors include weather patterns and economic disruptions.
Major Commodities and Their Prices
Several commodities dominate the market. Wheat, corn, soybeans, and rice represent major staples, with their prices showing variable trends.
- Wheat: Global wheat prices surged by 30% in the first half of 2023 due to droughts in major producing regions.
- Corn: Corn prices rose 15% in 2023, driven by increased demand for biofuels and adverse weather conditions.
- Soybeans: Soybean prices increased by 20% in 2023, influenced by strong demand from China and crop yield issues in South America.
- Rice: Rice prices remained stable in 2023, experiencing only a 2% increase, supported by robust production levels in Asia.
Impact of Global Events on Commodity Prices
Global events deeply affect agricultural commodity prices. Trade policies, pandemics, and geopolitical tensions are primary influences.
- Trade Policies: Tariff changes and trade agreements can cause significant fluctuations. For instance, the US-China trade war from 2018-2020 led to unpredictable soybean prices.
- Pandemics: COVID-19 disrupted supply chains, causing initial price spikes for various commodities. Recovery phases saw stabilization.
- Geopolitical Tensions: Conflicts, particularly in major agricultural regions, impact prices. The Russian invasion of Ukraine in 2022 notably increased wheat prices due to interrupted exports.
Understanding these variables helps stakeholders navigate the complex landscape of agricultural commodity pricing.
Forecasting Methods
Accurate forecasting methods are essential for predicting agricultural commodity prices. These methods help stakeholders make informed decisions based on data-driven insights.
Statistical Approaches
Statistical approaches rely on historical data to identify price trends. Techniques like moving averages, autoregressive integrated moving average (ARIMA), and regression analysis offer foundational insights.
- Moving Averages: Simplifies data by averaging price points over a specific period. This smooths out fluctuations, making it easier to identify trends. For example, a 30-day moving average helps spot long-term trends by reducing daily volatility.
- ARIMA: Combines autoregression, differencing, and moving averages. It’s used for time series data to predict future points. For instance, ARIMA models have successfully forecasted wheat prices by analyzing past data and identifying seasonality patterns.
- Regression Analysis: Examines relationships between variables. In agricultural pricing, it might correlate rainfall levels with crop yields. This helps estimate supply fluctuations and their impact on prices.
Machine Learning Models
Machine learning models offer advanced predictive capabilities through complex algorithms. These models learn from data, improving accuracy over time.
- Artificial Neural Networks (ANNs): Mimic the human brain’s neuron structure to process data. ANNs can detect intricate patterns in commodity prices, making them useful for complex forecasting tasks. For example, ANNs have been effective in predicting corn prices by analyzing numerous inputs like weather patterns and market demand.
- Support Vector Machines (SVMs): Optimize classification and regression tasks by finding the best boundaries between data points. SVMs excel in non-linear data prediction, making them ideal for volatile commodity markets.
- Random Forests: Use multiple decision trees to improve prediction accuracy. Each tree provides a different outcome, and the “forest” averages these results for a more reliable forecast. For instance, random forests have enhanced soybean price predictions by considering various factors like global trade and local production.
Incorporating these forecasting methods improves decision-making processes by providing precise and reliable price predictions.
Future Trends and Predictions
Rapid advances in data analytics and machine learning shape future trends in agricultural commodity prices. Accurate forecasting helps stakeholders react promptly to market shifts.
Short-term Price Forecasts
Short-term forecasts typically span up to one year. Predictive models, such as moving averages and ARIMA, use recent historical data to forecast imminent price movements.
For instance, the moving average method smooths out fluctuations by averaging prices over a specific number of periods. ARIMA (Auto-Regressive Integrated Moving Average) then combines differencing, autoregression, and a moving average model to capture temporal dependencies in price data.
Machine learning models like Support Vector Machines (SVM) and Random Forests play crucial roles in short-term forecasting. SVM finds a hyperplane in a high-dimensional space that classifies data points effectively, improving forecast accuracy. Random Forests aggregate multiple decision trees to enhance prediction robustness by reducing overfitting.
Long-term Market Outlook
Long-term forecasts extend beyond one year, addressing structural factors influencing prices. Analysts examine macroeconomic indicators, trade policies, and technological advancements.
Regression analysis evaluates relationships between independent variables (e.g., GDP growth) and agricultural prices to provide insights into long-term trends.
Advanced machine learning methods, like Artificial Neural Networks (ANN), use vast datasets to predict future scenarios. ANNs mimic human brain processes to recognize complex patterns in data.
Additionally, climate models predicting weather changes and their impact on crop yields are integral to long-term price forecasting.
By incorporating these sophisticated techniques, stakeholders gain a comprehensive understanding of future agricultural commodity prices, facilitating strategic planning and decision-making.